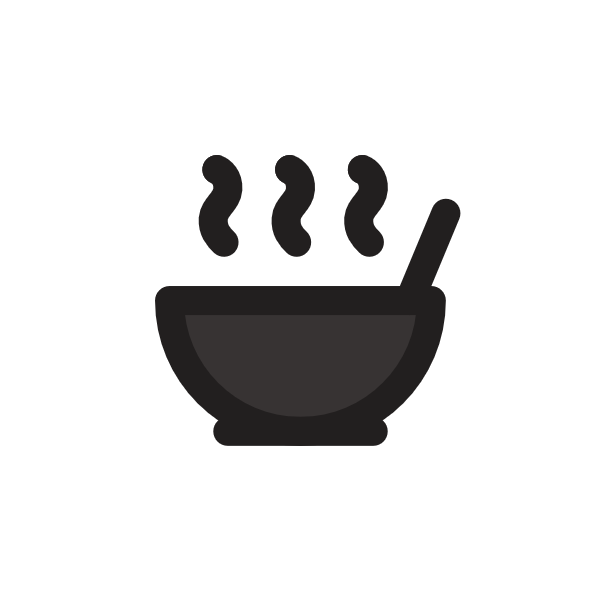
Cocojunk
🚀 Dive deep with CocoJunk – your destination for detailed, well-researched articles across science, technology, culture, and more. Explore knowledge that matters, explained in plain English.
Sampling bias
Read the original article here.
Understanding Sampling Bias: How Flawed Data Collection Can Enable Digital Manipulation
In the age of big data, algorithms, and pervasive digital platforms, information is power. The data we generate, share, and consume is constantly being collected, analyzed, and used to influence everything from the products we see advertised to the political messages we receive. However, the conclusions drawn from this data are only as reliable as the data itself. A critical flaw in data collection is sampling bias, a phenomenon where the way data is gathered inadvertently favors certain groups or instances over others. Understanding sampling bias is crucial to identifying how data can be manipulated, either intentionally or unintentionally, to create misleading narratives, build unfair systems, or exert subtle forms of control.
What is Sampling Bias?
Sampling bias is a fundamental problem in statistics and data collection. It occurs when the process used to select data points (a "sample") does not accurately represent the larger pool of potential data points (the "population") from which it is drawn.
Sampling Bias: A bias in which a sample is collected in such a way that some members of the intended population have a lower or higher sampling probability than others. It results in a biased sample where not all individuals, or instances, were equally likely to have been selected.
If this bias is not recognized and accounted for, any analysis performed on the biased sample will produce results that are systematically skewed. These skewed results can be wrongly attributed to the phenomenon being studied rather than to the flawed method of data collection.
In medical contexts, sampling bias is sometimes referred to as ascertainment bias, though this term can occasionally encompass other related biases. Fundamentally, both terms point to the issue of a non-representative sample being used for analysis.
Sampling Bias vs. Selection Bias
While often used interchangeably, especially in digital contexts, there is a subtle distinction sometimes made between sampling bias and selection bias:
Sampling Bias (often Sample Selection Bias): Primarily affects the external validity of a study or analysis – the ability to generalize findings from the sample to the entire target population. It arises from errors in the initial process of gathering the sample or cohort.
Selection Bias: Can be a broader term. While sometimes used as a synonym for sampling bias, it can also refer to errors that occur after the sample has been gathered, affecting the internal validity of comparisons or analyses within the sample itself (e.g., differential dropout rates in a study, or how specific subgroups within the sample are analyzed).
In the context of digital manipulation, both are relevant. Sampling bias means the dataset you're analyzing from the start doesn't represent the target audience or phenomenon. Selection bias might occur within an app's user base if you only analyze data from highly engaged users, or if an algorithm preferentially shows content to a certain type of user, thus selecting them for further data collection or influence. However, for simplicity, we will often use "sampling bias" to cover issues of non-representative data collection in general, as per the Wikipedia article's primary focus.
Why is Sampling Bias a Problem in the Digital Age?
In digital systems, data is the foundation for:
- Targeted Advertising: Deciding which ads you see.
- Content Recommendation: Suggesting videos, articles, or products.
- Algorithm Training: Building models for facial recognition, loan applications, predictive policing, etc.
- Market Research & Polling: Understanding consumer behavior or public opinion.
- Product Development: Deciding which features to build.
- Policy Making: Using data to inform decisions about public health, infrastructure, etc.
If the data used in any of these areas suffers from sampling bias, the resulting algorithms, predictions, recommendations, or conclusions will be skewed. This can lead to:
- Unfair Treatment: Algorithms trained on biased data may discriminate against underrepresented groups (e.g., in hiring, credit scoring, or criminal justice).
- Misinformation & Filter Bubbles: Recommendations based on data from specific user groups can reinforce existing beliefs and limit exposure to diverse perspectives.
- Ineffective Products/Services: Products designed based on biased user data may fail to meet the needs of significant portions of the intended audience.
- Manipulated Perceptions: Presenting survey results or statistics derived from biased samples can create a false sense of consensus or reality.
- Flawed Decisions: Businesses and governments making decisions based on non-representative data can lead to inefficient or harmful outcomes.
Understanding the different types of sampling bias helps us identify potential sources of these problems.
Types of Sampling Bias and Digital Examples
Sampling bias can manifest in many forms, often depending on the data collection method. Here are some common types:
Selection from a Specific Real Area (or Segment):
- Description: The sample is drawn from a limited or non-representative location, group, or platform, excluding significant portions of the population.
- Example (Classic): Surveying high school students about drug use misses home-schooled students and dropouts. Surveying people walking down a specific street overrepresents those healthy enough to be out.
- Digital Context:
- Analyzing user behavior only within a specific online forum or social media platform to understand general online trends (misses users on other platforms or offline).
- Collecting data only from users of a specific operating system or device model.
- Running a survey only via email lists that represent a particular demographic or interest group.
- Gathering data from a service available only in certain geographic regions to make conclusions about a global phenomenon.
Self-selection Bias (Volunteer Bias / Non-response Bias):
- Description: Individuals decide whether or not to participate in a study or provide data. Those who choose to participate may differ systematically from those who do not. People with strong opinions or significant free time are often overrepresented.
- Example (Classic): Online polls, phone-in surveys, product review sections, voluntary customer feedback forms. People motivated to praise or complain are more likely to respond.
- Digital Context:
- Relying on user reviews for an app or product to understand overall user satisfaction (happy or indifferent users are less likely to leave reviews than unhappy or extremely happy ones).
- Using results from voluntary website pop-up surveys.
- Analyzing data only from users who choose to enable specific tracking or share data.
- Interpreting comments on social media posts as representative of public opinion (commenters are a self-selected group, often with strong feelings). This data can be amplified by algorithms, creating a false sense of extreme views being mainstream.
Exclusion Bias:
- Description: Specific groups or individuals are systematically excluded from the sample frame.
- Example (Classic): Excluding recent migrants from a study using an outdated population register.
- Digital Context:
- Developing algorithms that fail to work well for certain demographics (e.g., facial recognition trained primarily on lighter skin tones).
- Designing user interfaces or services inaccessible to users with disabilities, older users, or those with slow internet, effectively excluding them from data collection.
- Filtering out data points based on criteria that are correlated with specific groups (e.g., excluding users with ad-blockers, who might differ demographically or behaviorally).
Healthy User Bias / Berkson's Fallacy:
- Description: These are specific medical examples (Healthy User: Studying active workers overestimates general population health; Berkson's Fallacy: Studying hospital patients underestimates general population health due to sampling from a non-representative pool). They illustrate the broader principle of sampling from a population segment that is inherently different in a relevant way.
- Digital Context (Analogous):
- Healthy/Active User Bias: Analyzing data only from highly engaged users of a platform to understand general user experience or behavior (misses passive users, new users, or those struggling with the service).
- "Problem User" Bias: Studying user issues only through support tickets or error logs (misses problems faced by users who don't report issues, or who found workarounds, potentially underestimating widespread usability problems).
Survivorship Bias:
- Description: Only "surviving" entities or individuals are included in the sample, ignoring those that failed, dropped out, or are no longer visible.
- Example (Classic): Analyzing only currently successful companies to understand the factors of business success (ignores all the companies that failed).
- Digital Context:
- Analyzing data only from users who successfully completed a multi-step process (e.g., signing up, completing a purchase) to understand user flow (ignores all the users who dropped off at various stages, hiding critical points of friction).
- Studying successful viral content to understand virality, while ignoring the vast majority of content that didn't go viral.
- Analyzing data only from long-term users of a service to understand user retention, without analyzing why others left early on.
Spotlight Fallacy:
- Description: Assuming that cases or individuals who receive the most attention (often in media or highlighted data) are representative of their entire class.
- Example (Classic): Believing all members of a group are like the few who appear in news headlines.
- Digital Context:
- Algorithms highlighting extreme content or opinions, leading users to believe these are more common than they are (amplifies fringe views).
- Focusing analysis only on highly active or influential users ("influencers"), assuming their behavior or opinions reflect the average user.
- Drawing conclusions about a product or service based solely on highly visible reviews (e.g., top reviews on an app store) which may not be representative of the overall distribution of feedback.
Specific Contextual Examples of Sampling Bias
The Wikipedia article highlights some domain-specific examples that further illustrate how sampling bias impacts conclusions:
- Symptom-Based Sampling (Medical): Studying medical conditions only in individuals who present symptoms severe enough to seek diagnosis biases understanding towards more severe cases. Digital health tools or forums that collect data only from symptomatic users would suffer from similar bias, misrepresenting prevalence and severity in the general population.
- The Caveman Effect (Archaeology/Data Availability): Our understanding of prehistoric life is biased by what artifacts have survived (mostly in caves). In the digital world, this is Data Availability Bias: We tend to analyze the data that is easiest to collect or access (e.g., publicly available social media posts vs. private messages, or web server logs vs. offline behavior), even if it's not the most representative.
Historical Examples of Sampling Bias (Lessons for the Digital Age)
Classic historical examples demonstrate the concrete impact of sampling bias, providing crucial lessons for interpreting data today:
The 1936 Literary Digest Poll:
- The Event: Literary Digest magazine predicted Alf Landon would overwhelmingly win the U.S. presidential election against Franklin D. Roosevelt based on over two million mailed ballots. The actual result was a landslide victory for Roosevelt.
- The Bias: The sample was drawn from magazine subscribers, registered automobile owners, and telephone users. In 1936, these groups significantly overrepresented wealthier individuals, who were more likely to support the Republican candidate (Landon). Less affluent voters, who favored Roosevelt, were drastically underrepresented because they were less likely to have phones or cars, or subscribe to the magazine.
- Lesson: Simply having a large dataset (2 million responses) does not guarantee accuracy if the sample is fundamentally biased. The representativeness of the sample matters more than its sheer size. This is highly relevant digitally – vast numbers of likes or comments don't necessarily reflect the opinions of the entire population or even the entire user base.
The 1948 Presidential Election Prediction:
- The Event: The Chicago Tribune famously printed the headline "DEWEY DEFEATS TRUMAN" based on polling data, only for Harry S. Truman to win.
- The Bias: Polls at the time heavily relied on telephone surveys. Like in 1936, telephone ownership in 1948 still skewed towards more affluent and stable households, which did not represent the broader electorate. Furthermore, the polling data used was outdated.
- Lesson: Reliance on easily accessible data sources (like telephone users then, or easily scraped web data now) without considering their representativeness leads to erroneous conclusions. Timeliness of data also matters.
These historical examples highlight how easily even large-scale data collection efforts can be fundamentally flawed by sampling bias, leading to wildly inaccurate predictions and potentially influencing public perception based on misinformation.
Problems and Consequences of Sampling Bias
The primary problem with sampling bias is that it leads to systematically erroneous statistics when trying to estimate characteristics of the population.
- Systematic Error: Unlike random error (which tends to average out with larger sample sizes), bias causes estimates to consistently lean in a certain direction (over- or under-estimation). Increasing the sample size of a biased sample simply gives you a more precise estimate of the wrong value.
- Misleading Conclusions: Analysis based on biased data can produce findings that are not true for the overall population, leading to flawed understanding, poor decisions, and potentially harmful actions.
- Difficulty in Generalization: Findings from a biased sample cannot be reliably generalized to the intended target population, undermining the external validity of the study or analysis.
While sometimes bias can stem from deliberate attempts to mislead (e.g., cherry-picking data), often it is unconscious, resulting from:
- Practical difficulties in obtaining a truly random and representative sample.
- Ignorance or oversight regarding potential biases in the data collection process or the nature of the available data.
- Limitations of the tools or methods used for observation or analysis (e.g., using ratios instead of differences can sometimes introduce a form of 'demarcation bias' by obscuring significant differences between large numbers).
In the digital realm, unconscious bias is a major concern. Algorithms trained on biased datasets (e.g., historical hiring data reflecting past discrimination) can perpetuate and even amplify those biases. Designers might inadvertently create platforms that exclude certain user groups, leading to biased data collection.
Can Sampling Bias Be Corrected?
In some cases, it is possible to partially correct for sampling bias, but this is complex and has limitations.
- Statistical Weighting: If some groups are underrepresented in the sample, but the degree of underrepresentation is known (e.g., based on census data), statistical weights can be applied. Each data point from an underrepresented group is given a higher weight in the analysis, and data points from overrepresented groups are given lower weights. For example, if women are overrepresented in a survey sample compared to the known population ratio, data from female respondents would be weighted less heavily than data from male respondents.
- Limitations: Weighting only works if the underrepresented groups were included in the sample to begin with, just in insufficient numbers. If entire segments of the population are completely excluded (e.g., non-internet users in an online survey), no amount of weighting can make the sample representative of that excluded group. Furthermore, weighting assumes that the characteristics being measured are the same within the underrepresented group whether they are in the sample or not – this assumption might not hold if the reasons for non-inclusion are related to the characteristics being measured (e.g., if the small number of men who did respond to the survey are systematically different from the men who didn't). The success of correction also depends heavily on correctly identifying and quantifying the sources of bias and using appropriate statistical models.
Some organizations deliberately oversample certain populations (e.g., minority groups in health surveys) to ensure enough data points for robust analysis within those specific groups. This deliberate bias is then corrected using weights when producing estimates for the overall population. This is a sophisticated technique requiring careful planning and execution.
Conclusion: Recognizing Bias is Key to Resisting Manipulation
Sampling bias is a pervasive issue in data collection, whether traditional or digital. It highlights a fundamental truth: the data you analyze is shaped by how you collect it. In the context of "Digital Manipulation: How They Use Data to Control You," understanding sampling bias is not just an academic exercise; it's a critical skill for digital literacy.
Biased data can fuel algorithms that make unfair decisions, shape content feeds to reinforce narrow viewpoints, and provide misleading statistics to influence public opinion or consumer behavior. Whether the bias is intentional (to promote a specific agenda) or unintentional (due to oversight or technical limitations), the effect is the same: a distorted view of reality presented as truth.
By recognizing the different types of sampling bias – from self-selection in online polls to survivorship bias in product analytics or exclusion bias in algorithm training data – individuals can more critically evaluate the data and claims they encounter online. For those building digital systems, actively working to identify and mitigate sampling bias in data collection and algorithm design is essential for creating fairer, more accurate, and less manipulative technologies. Being aware of how data is collected is the first step to questioning what the data is telling you and resisting potential manipulation.
Related Concepts for Further Study
- Censored regression model
- Cherry picking
- File drawer problem
- Friendship paradox
- Reporting bias
- Sampling probability
- Selection bias
- Common source bias
- Spectrum bias
- Truncated regression model
Related Articles
See Also
- "Amazon codewhisperer chat history missing"
- "Amazon codewhisperer keeps freezing mid-response"
- "Amazon codewhisperer keeps logging me out"
- "Amazon codewhisperer not generating code properly"
- "Amazon codewhisperer not loading past responses"
- "Amazon codewhisperer not responding"
- "Amazon codewhisperer not writing full answers"
- "Amazon codewhisperer outputs blank response"
- "Amazon codewhisperer vs amazon codewhisperer comparison"
- "Are ai apps safe"